Machine-Learning Algorithms Can Recognize Rare Dementias Such as FTD, Study Finds
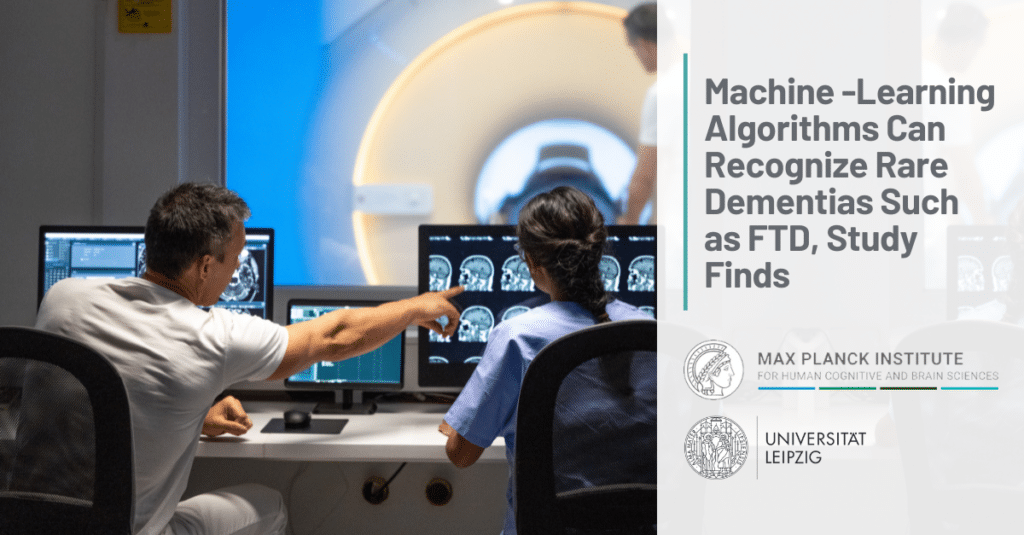
A study published in the Neuroimage: Clinical medical journal found that machine-learning algorithms are capable of recognizing patterns of atrophy associated with rarer neurodegenerative diseases such as FTD.
Conducted by researchers at the Max Planck Institute for Human Cognitive and Brain Sciences and the University of Leipzig Medical Center in Germany, the study was intended to address the considerable obstacle of obtaining a correct diagnosis of less common brain disorders. When diagnosis is uncertain, treatment becomes difficult because medical professionals don’t know which therapies to pursue to properly address someone’s symptoms.
“Questions such as those posed by [patients] in our study are typical in everyday clinical practice,” study co-author Matthias Schroeter told Neuroscience News. “First and foremost, the question arises as to the correct diagnosis so that the therapy can be adapted to each individual patient and their specific disease.”
To evaluate machine learning-algorithms as a tool for improving diagnosis, researchers used artificial intelligence to parse imaging data from 426 participants with a form of dementia (including several disorders in the FTD family) and data from 51 control participants. The machine-learning model examined 64 different regions of the brain, checking the volumes of different types of brain tissue for atrophy.
According to researchers, one machine-learning model could classify the correct type of dementia with an accuracy range of 71-95%. A more complex machine-learning model had greater accuracy, but still fell below 100%. Researchers noted that the more complex model did not have the same performance for all types of dementia, and was better at classifying disorders with atrophy patterns that focused on a certain region of the brain.
While machine learning has its limitations, algorithms can still be beneficial in reducing the time to diagnosis, according to the authors of the study.
“Even if the course of these diseases progresses, those affected in early stages of the disease can continue to work and manage their daily lives with support. This is why early diagnosis and individual adaptation of therapy measures are crucial,” Schroeter said.
Interested in reading more about the use of technology like machine learning to diagnose FTD? Check out this article about the use of wearable sensors to monitor the progression of progressive supranuclear palsy, or this article about a study that utilized machine learning to investigate a 120-year-old theory on dementia.
Are you interested in participating in a study like the one you read about? Check out AFTD’s Studies Seeking Participants page.
By Category
Our Newsletters
Stay Informed
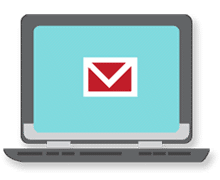
Sign up now and stay on top of the latest with our newsletter, event alerts, and more…